AI Tool from Stefanini, Lawrence Tech Helps Run Smart Factories
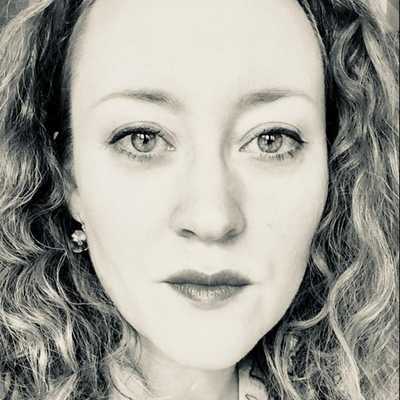
By Laura Cowan
Laura K. Cowan is a tech editor and journalist whose work has focused on promoting sustainability initiatives for automotive, green tech, and conscious living media outlets.
Sophie is an AI virtual assistant that integrates with business intranets to answer employee questions.
AI tool Sophie, from Stefanini, is now helping run factories, because of a partnership between Stefanini Group and Lawrence Technological University (LTU). It's one of the first applications we've seen of natural language processing for AI integration with smart manufacturing, and we sat down with Stefanini leadership to learn about the challenges of developing AI for manufacturing applications and why this type of application is a cutting edge use of the technology.
Sophie is a virtual artificial intelligence (AI) assistant developed by Stefanini, a $1 billion global technology company specializing in digital solutions with an office in Metro Detroit and locations around the world. Their AI tool Sophie has been used so far to create a natural language processing AI tool for retrieving information about businesses. This is the first time the company has branched out into a partnership with manufacturing.
LTU students and faculty are teaching Sophie how integrate into smart manufacturing environments on LTU's campus with self-adaptation and contextual automation, made possible by Stefanini partner, Rockwell Automation. The students, who are part of a mentorship program run by Stefanini to help young people get started with careers in AI, are working with Sophie to design everything from architectural design to analyzing Sophie’s mind map flow chart.
“We began partnering with Lawrence Tech about three years ago and we’re thrilled how our collaboration efforts have grown,” said Fabio Caversan, vice president of digital business and innovation at Stefanini. “Using natural language and machine learning, Sophie can simplify interactions with complex systems like smart manufacturing environments. LTU students have played a critical role in making this integration process as smooth as possible.”
Designed with an original set of artificial intelligence algorithms and replacing script-based human service with automation, Sophie is already trained in voice processing, text interpretation and self-learning initiation and can be used for various business applications to find and process information. But how to integrate that with an entirely separate manufacturing software and create a simple tool? How do you train an AI model on manufacturing data and know that you're succeeding?
Stefanini says Sophie can achieve the same results as other AI technologies with five percent of the training required by other tech tools, and that's because it's not the standard model of AI that requires millions of data points to learn how to predict outcomes.
"This AI component we integrated with the platform [for the] smart manufacturing landscape is a component we've been working on for 10 years," Caversan explains, "so it actually started back when Stefanini acquired a small startup in Brazil that was researching natural language processing technology. Since the beginning, our approach to natural language technology was something that can be simple and explainable. We are using technology that's different from the market, which has been using AI models that require a lot of data to be trained.... We use a more semantic approach so it recognizes meaning, and makes connection between concepts. It's not just we that have this anymore, there is a trend toward that in the market, but [through this technology] we can teach relationship and meaning to the AI model and then with just a few examples it can answer a question from the user."
So natural language processing can create integrated systems that allow a simpler training process. How simple are they for the end user?
"From the application perspective," Caversan says, "the goal has always been to put a human-like interaction on top of a system that is complicated to operate. So let's say [to get a certain answer or result] you have to go to 10 different screens and click here and type there, but if you gave that instruction to a human you might just give a single sentence of instruction. That's the goal: to put the natural language layer on top of complex systems. We've been doing that for all this time in applications on the software side. What we realized that has a lot of value is that when you have an operator of a complex plant, he has huge systems with all this equipment and these measurements. These guys are skilled, so they can get to what they desire, but that doesn't mean it's simple or quick. If I ask a simple sentence question of this AI tool, I could use this on Teams or SMS text, and I can get that answer immediately."
"How can you map all those machines and elements to a conversational interface? Well, there's some work in there," Caversan says, "but we've always been working with meaning instead of millions of examples, and that made it easier. If you have motors [you want to work with] and [we show the AI] this is one example of a motor and that's it, whenever this is a question related to a motor, they are semantically related."
But how do you know you're succeeding? Even with the simplicity of a natural language processing model that doesn't require so many data points to train, how do you know where the holes are in the training?
"[If you have] huge data sets and they are behaving, you might get 80% accuracy in models that use lots of data points. When you have an answer that's not what you want, you have to add more examples and retrain everything and hope you get your answer and you're not messing up previous answers. You have huge data sets divided between what you're using to train and using to validate. Our model is not a black box, it's a white box," Caversan explains. "Whenever you get an answer, you can open a simulation and ask why did you give me that answer and get the connections between the concepts and how you got the answer. You can see there's a relation here that's wrong or not strong enough, and you can remove that. You're not only helping that particular case, but you're helping all the other questions related to that semantic task to reach a conclusion for the user."
Surely there are challenges layering natural language processing on top of complex systems and integrating them with other complex software, though. Caversan says the challenge is in integrating with other systems, because you have to mine all the data you have available from that other system you're integrating with in order for the natural language processing to benefit from it.
"The major challenge in this project -- which might be the reason we don't see a lot of applications in manufacturing yet -- is to close that gap between systems in manufacturing to this modern software development."
To learn more about Stefanini Group, please visit www.stefanini.com and for more information about Lawrence Technological University visit www.ltu.edu.
ai tools, ai tools for manufacturing, custom sofware, lawrence tech, natural language processing, smart manufacturing, sophie, stefanini